August 12, 2022
August 12, 2022

Taken from the post on More Intelligent Tomorrow by DataRobot.
Banking is on the cusp of an AI revolution that could prove transformative. According to McKinsey & Company, disruptive AI tech can boost profits, lower costs through efficiencies, aid rapid innovation cycles, and improve and deepen customer relationships. Banks that don’t go AI-first will “risk being overtaken by competition and deserted by customers.”
With that in mind, how many are taking advantage? Business Insider reveals that 80% of respondents are “highly aware of the potential benefits presented by AI and machine learning,” and 75% of those at banks with over $100bn in assets say they are already implementing AI strategies, although that figure falls to 46% for those with assets below $100bn.
Notably, the industry is also more bullish than most. In DataRobot’s AI and the Power of Perception survey from June 2022, respondents from banking and finance were more likely than the global average to think AI would be a major game changer over the next five years – and less likely to fear the tech and be concerned about implementation.
To dig deeper, we spoke directly to industry experts about the best ways to use AI and machine learning in banking, including how to understand the challenges, deal with risks, and define paths to success.
Here’s what you need to do:
1. Use data and AI to enrich and personalize the customer experience

Don’t just use tech and data to understand risk and create dashboard views – have it boost efficiency and improve the customer journey.
If a customer’s been with a bank for ten years, should they really need paperwork to verify income when applying for a mortgage?” asks Joseph Burdis, Lead Data Scientist at DataRobot.
Along with getting people where they need to be more rapidly, data and insights could improve the customer experience through personalization. “Behavior analysis and predictive suggestions will enable banks to drive value through giving support at the right time,” says Claire May, UK Head of Business & Change Management, Corporate and Commercial Banking at Santander UK.

This improvement in customer experience, thinks Claire, will make “navigating the world of finance simpler,” notably during pivotal moments like buying a home. But she says SMEs would benefit too: “The right input at the right time could supercharge a business to scale up and take advantage of opportunities. That would maximize value for the bank and customer alike.”
2. Unlock new opportunities with astute data use
Every purchase, transfer, and payment is a data point – and the sheer volume of data brings opportunities, thinks Scott Munson, Director, Data Science & Analytics at Evolutio: “Even the smallest financial institutions have a treasure trove to translate into business value. They just need clear use cases with business value and vision to make it happen – and drive to push through organizational barriers.”

HP Bunaes, Executive Director, AI & Machine Learning at JPMorgan Chase & Co., agrees. He considers credit a “deep well of opportunity.” With better credit models and more data, he says banks can rely less on traditional information sources and benefit through innovation:
Credit scores don’t tell you much that other banks don’t already know. So find opportunities your competitor is not able to spot.”
This type of innovation might require you to take more risk, but “you do it safely, because you’ve figured out unique features that are informative, or how to reach a different set of clientele underserved by a particular product,” HP adds
3. Start small and prioritize speed
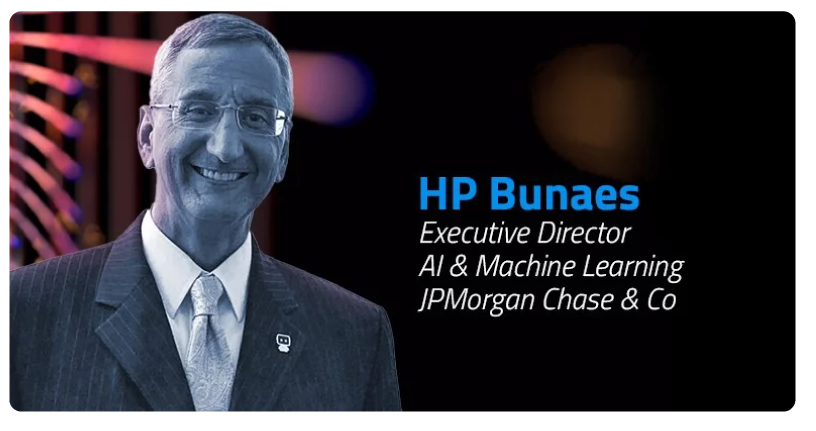
HP says having a business perspective is vital when looking into AI in banking: “Think where you’ll get value and meaningfully impact on business metrics, and where you have data to make everything feasible – without a massive year-long data project that will cost millions before it can do anything.”
Scott agrees, noting that starting the process and moving to production are the hardest parts of an AI/ML project. So he says to “start small, learn by doing,” leverage a service partner if you don’t have relevant in-house talent, and put models in production, so they deliver real value. “This approach is so much faster and more effective than multi-year initiatives,” he adds.
If you have too many ideas, HP suggests you “start with highest value/lowest complexity use cases.” Those you think will be the most transformative have the highest execution risk and low chances of delivering. Low-hanging fruit might also mean lower value – but you have a better chance of delivering.
4. Get the right people in place – at every level
According to Scott, people are the limiting or enabling factor in AI/ML projects: “Success is dictated by bringing together the right stakeholders, defining clear goals, leveraging the right technology, and the drive of a few key players with the vision to complete the work.” Once your first project is done, he says to share what you’ve achieved, learn from it, and do more projects with a broader group.
HP says an area where banks struggle is in getting the right people fully on board:
In many cases, executive management delegates development of analytics to data scientists and tech people, sits back, and hopes good things happen. I’ve seen banks try to do sophisticated things with enormous amounts of data and the best data scientists. Then they’re disappointed they don’t quickly get incredible results.”
The solution, explains HP, is to take more care with application and direction. Leave data scientists to pick what they want to work on and they’ll “pick the coolest, sexiest stuff every time.” So executives have to be involved – and that starts with picking the right applications of AI and machine learning.
5. Be aware that legacy thinking and platforms are blockers
Aside from people, legacy tends to be the biggest blocker to success and agility in banking when it comes to AI/ML projects. “Much of the industry operates with multiple platforms,” says Claire.
Data can be hard to organize and access without a great deal of effort, which can make utilizing or implementing AI/ML a challenge.”
According to Joseph, much of this stems from banking’s history of “managing data in pockets” rather than it being integrated, due to customer data being highly protected – for good reason. He adds that model governance adds further barriers to rapid adoption of AI/ML.
When approaching projects, it’s important to understand your starting point as much as your intended destination, how to get the fullest view of data, and how to wrangle said data for best effect.
6. Design for fairness, because regulation is coming
With AI/ML increasingly impacting individuals (rather than just corporate entities), fairness is a major consideration. “There will be more sensitivity toward societal impact and the embedded bias in using data based on decades of biased – albeit unintentionally so – decision making,” thinks HP.
This means “explainability” will become a really big deal, not least in banking and finance.
If you make a decision that adversely affects a client – like declining to extend credit – you’ll have to explain why,” says HP.
And he means not only explaining to the customer, but also to the regulators — local and global.
In short, you must be able to defend your models and make sure they’re as fair as possible. Don’t do this and regulation will eventually force your hand; you’ll then have to spend time and money dealing with something that should have been dealt with right from the start.
7. Focus on what happens after you deploy – not just before
It’s not enough to put an AI/ML project into production and do nothing more. “Sometimes banks will have a model that was developed and trust it’s working,” says Joseph. “But they need to be constantly evolving and redeveloping it.”
HP says this problem is only going to get worse: “As we move from dozens to thousands of models, it becomes harder to manage complexity. As models become ubiquitous, your operational risk goes up.” Echoing Joseph, he states that you
don’t need to know a model was good when deployed, but that it’s good and still working now.”
The solution is data management: make sure you know what AI/ML models you have, the data they use, who’s using them and what they’re doing with the results. That way, you can mitigate problems that might occur from things you didn’t anticipate on day one – which will only grow with the number of deployed models and increased interconnectedness of data.
8. Get leadership on board – and have them act now
Business leaders need to be educated and aware of AI/ML – and it’s vital they lead, not follow. HP says they need to know what AI/ML is capable of and spot good use cases. It’s important they understand what their analytics people are working on and why. And they must drive priorities and innovation, along with making sure business heads take rollout, adoption, training, and communicating seriously.
If all these things are not on a CEO’s agenda, that’s a problem,”
he warns. “It’s too strategically important and potentially transformative.” But those leaders that do this and get things right, thinks HP, will find their organizations separate from the pack in terms of tangible business performance: “They’ll innovate faster and get solutions implemented more rapidly.”
Conversely, those banks and financial institutions that take a “wait and see” approach – or whose executives don’t take AI/ML seriously or don’t think strategically about the opportunities – will find themselves in a situation where they just can’t catch up.
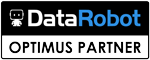
Evolutuio Data Science & Analytics: Every business is on a journey delivering value from their data.